Revolutionizing Video Labeling with Machine Learning Tools
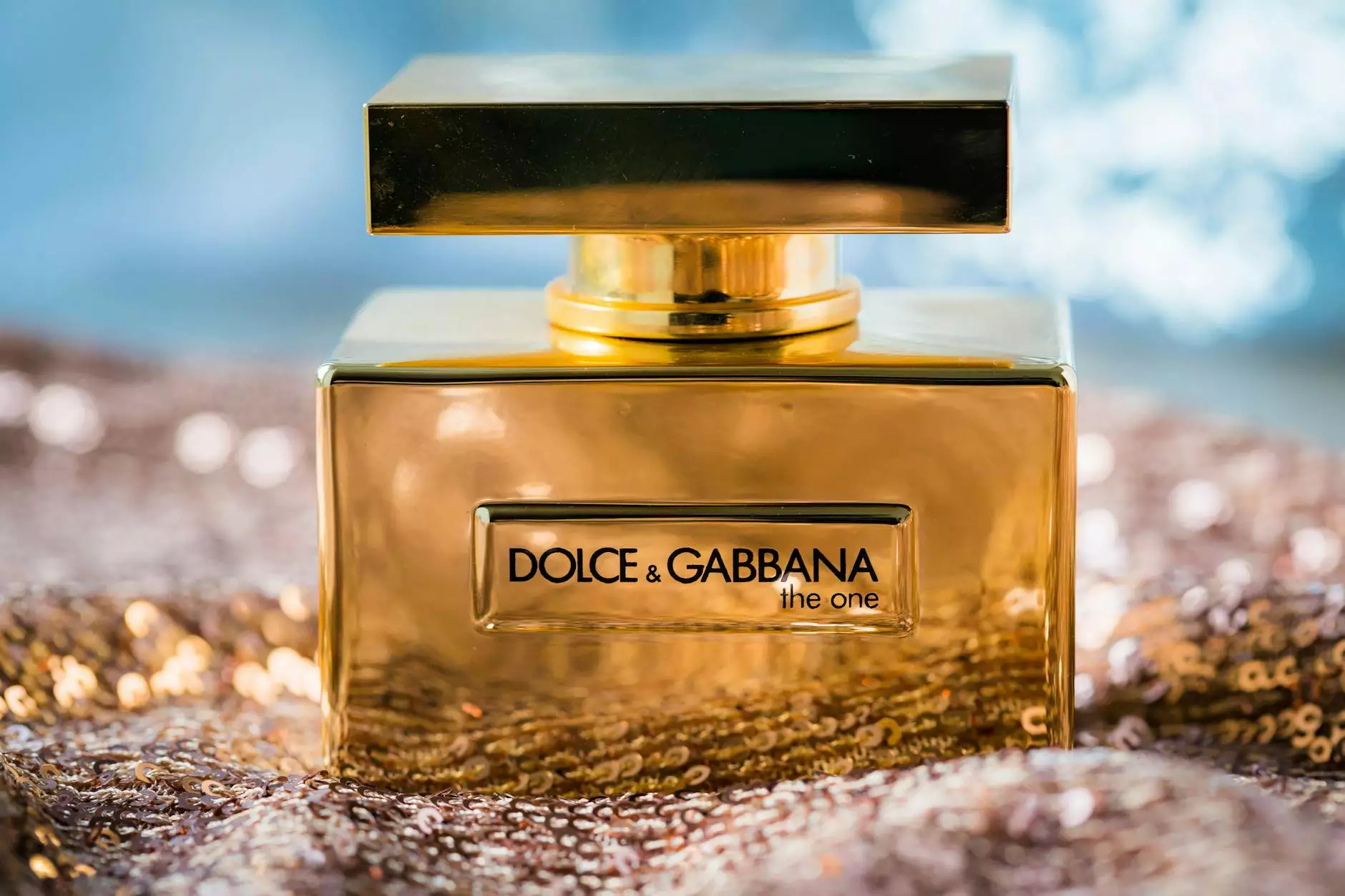
In the fast-evolving world of artificial intelligence and machine learning, the need for precise and efficient data labeling tools has become paramount. Among these, video labeling tool machine learning solutions stand out as vital resources for businesses seeking to leverage video data for various applications, ranging from autonomous vehicles to surveillance systems. In this article, we will delve into the significance of video labeling tools, their applications, and how they integrate machine learning to facilitate accurate data annotation.
Understanding Video Labeling Tools
Video labeling tools are specialized software applications designed to annotate and classify various elements within video content. These tools empower organizations to prepare video data for machine learning models by accurately identifying and marking objects, actions, and events. The advent of machine learning has revolutionized the way these tools function, enabling automation and enhancing efficiency.
The Importance of Data Annotation in Machine Learning
Data annotation is a fundamental step in training machine learning models. It involves the process of labeling data so that algorithms can learn to recognize patterns and make predictions. In the context of video data, this can mean identifying objects in a scene, detecting actions, or segmenting different components of a video frame. There are several reasons why precise data annotation is crucial:
- Improved Model Accuracy: Correctly labeled data directly influences the performance of machine learning models. If the training data is inaccurate, the model's predictions will be unreliable.
- Time Efficiency: Automated video labeling tools save time compared to manual labeling, allowing businesses to quickly prepare their datasets.
- Scalability: Machine learning applications often require vast amounts of data. Video labeling tools help organizations scale their data annotation efforts effectively.
- Enhanced Understanding: Properly annotated video data fosters a deeper understanding of the content, enabling better insights and analytics for businesses.
How Machine Learning Enhances Video Labeling Tools
Integrating machine learning into video labeling tools transforms the way data annotation is conducted. Here are some key ways in which machine learning enhances these tools:
1. Automation of the Annotation Process
Machine learning algorithms can automatically identify and label objects within video frames, significantly reducing the need for manual input. This automation not only speeds up the annotation process but also minimizes human error, leading to higher-quality labeled data.
2. Continuous Learning and Improvement
Machine learning models continuously learn from new data. As more videos are annotated using these tools, the algorithms become better at identifying and labeling similar content in future videos. This capability fosters a cycle of improvement, where the quality of annotations increases over time.
3. Handling Complex Data Structures
Videos are inherently complex due to the multitude of moving elements and varying contexts. Machine learning excels at processing and understanding these complexities, enabling accurate and nuanced labeling of objects and actions in videos.
4. Improved Collaborative Features
Many machine learning-driven video labeling tools incorporate collaborative features that allow teams to work together seamlessly. Real-time updates and cloud-based solutions facilitate an efficient workflow, making it easier to manage large-scale video datasets.
Applications of Video Labeling Tool Machine Learning
The applications of video labeling tools powered by machine learning are vast and varied across multiple industries. Here are some notable examples:
1. Autonomous Vehicles
In the automotive industry, video labeling is essential for training AI systems to recognize pedestrians, obstacles, and traffic signals. Accurate labeling of these elements in training datasets is crucial for the safe operation of self-driving cars.
2. Security and Surveillance
Security systems utilize video footage to monitor activity and detect anomalies. Machine learning-driven labeling tools enable the identification of suspicious behavior, improving incident response and overall safety.
3. Sports Analytics
In sports, video analysis is used to enhance player performance and make strategic decisions. Machine learning tools can label plays and movements, providing coaches with valuable insights into game performance.
4. Healthcare
In the healthcare sector, video labeling tools assist in monitoring patients and surgical procedures. Machine learning can be leveraged to identify key events in a surgical video, providing feedback and enhancing training.
Choosing the Right Video Labeling Tool
When selecting a video labeling tool that incorporates machine learning, organizations should consider the following factors to ensure they choose the best solution for their needs:
1. Accuracy of Annotations
The primary objective of any video labeling tool is to provide accurate and reliable annotations. Research the tool's performance metrics and user reviews to ensure it meets your standards.
2. User-Friendly Interface
A user-friendly interface facilitates a smoother workflow, making it easier for team members to navigate the tool, especially when collaborating across various roles.
3. Scalability
Choose a labeling tool that can scale with your organization’s needs. As your dataset grows, the tool should be able to handle an increasing volume of video content without compromising performance.
4. Integration with Existing Systems
Ensure that the labeling tool can integrate seamlessly with your existing data management or machine learning systems. Compatibility can streamline your workflow and enhance productivity.
5. Support and Training Resources
Adequate support and training options are essential for onboarding teams to use new tools effectively. Ensure the provider offers robust documentation and support services.
Benefits of Implementing a Video Labeling Tool
The implementation of a video labeling tool powered by machine learning delivers multiple benefits to organizations, including:
- Increased Efficiency: Automated processes lead to faster annotation times, allowing teams to focus on analysis and application rather than repetitive tasks.
- Higher Quality Data: Improved accuracy in labeling directly translates to better model performance, enabling more reliable insights and decisions.
- Cost Reduction: By minimizing manual labor and accelerating the annotation process, organizations can reduce costs associated with data preparation.
- Business Growth: Enhanced data capabilities empower organizations to innovate, leading to competitive advantages in their respective industries.
Future Trends in Video Labeling Tools
As technology continues to advance, we can expect several trends to shape the future of video labeling tools in conjunction with machine learning:
1. Enhanced AI Capabilities
The evolution of AI will lead to even more sophisticated video labeling tools capable of understanding context and meaning, elevating the quality of annotations.
2. Real-Time Annotation
Emerging technologies may enable real-time video annotation, allowing immediate feedback and decision-making during recording sessions, benefiting industries like healthcare and sports.
3. Improved Collaboration Tools
Future tools will likely focus on enhancing collaboration features, enabling teams to work together more effectively across dispersed locations.
4. Accessibility Through Cloud Solutions
Cloud-based video labeling tools will increase accessibility, allowing users to access their datasets and tools from anywhere, fostering a more flexible work environment.
Conclusion
In conclusion, the integration of video labeling tools powered by machine learning is transforming the way organizations handle video data annotation. By enabling faster, more accurate, and scalable labeling processes, these tools are paving the way for advancements in various industries. Embracing this technology is essential for businesses looking to harness the full potential of their video data and maintain a competitive edge in today's data-driven landscape. At Keylabs.ai, we are committed to providing top-tier data annotation platforms that meet the evolving needs of our clients. By investing in sophisticated video labeling tool machine learning capabilities, businesses can drive innovation and growth in an ever-competitive market.