Mastering the Art of Labeling Training Data with Keylabs.ai
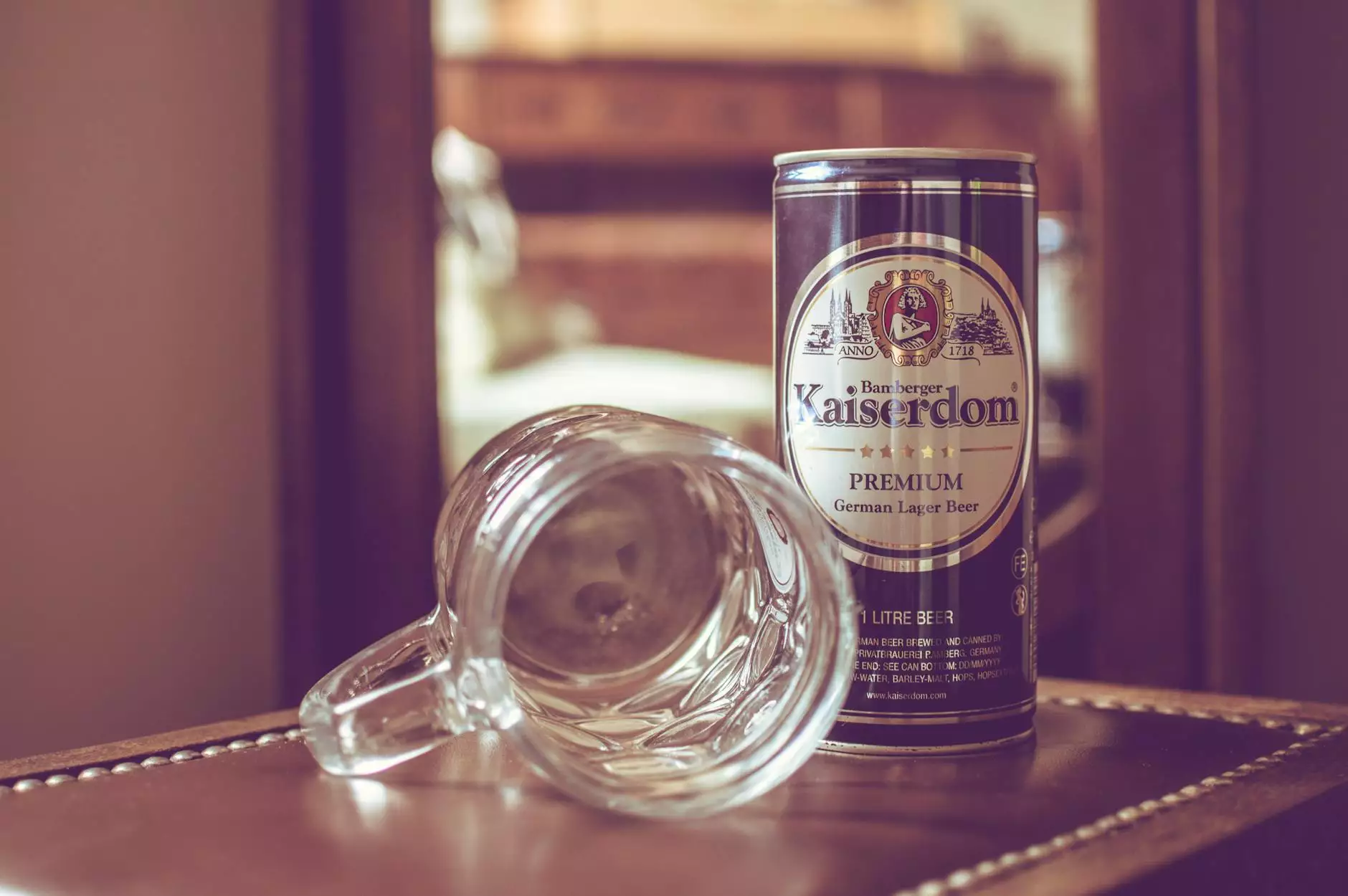
In the realm of artificial intelligence and machine learning, the concept of labeling training data plays a pivotal role in developing robust AI models. At Keylabs.ai, we understand that the quality and accuracy of labeled data significantly influence the effectiveness of machine learning algorithms. In this comprehensive guide, we will explore the intricate details of effective data annotation, the tools required, and how to leverage our platform to maximize your data's potential.
Understanding the Importance of Labeling Training Data
Labeling training data is not just a routine task; it's a critical process that lays the foundation for successful AI implementations. Here’s why it matters:
- Model Accuracy: Properly labeled data ensures that machine learning models can learn from accurate examples, leading to higher predictive accuracy.
- Reduction of Errors: Quality annotations help reduce biases and errors in model predictions, creating more reliable outcomes.
- Improved Decision-Making: When training data is well-labeled, models can provide insights that empower decision-making across various sectors.
- Scalability: The better the data is annotated, the easier it is to scale AI solutions as they rely on a more robust dataset.
The Process of Labeling Training Data
Understanding the workflow of labeling training data can enhance your capacity to manage data projects effectively. Here’s a detailed look at the process:
1. Data Collection
The first step in the journey of labeling training data is the collection of raw data. This can encompass various formats such as images, text, audio, and video. The richness and diversity of your data set will directly influence the training process.
2. Preprocessing Data
Raw data requires preprocessing to ensure it's ready for annotation. This involves cleaning data, removing inconsistencies, and converting it into a suitable format for labeling. Preprocessing is crucial as it helps eliminate noise that could mislead the AI during its training phase.
3. Annotation Guidelines Development
To achieve consistency in your labeling, develop comprehensive annotation guidelines. These guidelines provide instructions to annotators on how to categorize or tag the data accurately. Clear guidelines lead to greater accuracy and uniformity in annotations.
4. Labeling the Data
This is the core activity where data is annotated according to the defined guidelines. Various techniques can be utilized, including:
- Manual Annotation: Human annotators go through the data and apply labels. This method is typically used for complex datasets.
- Automated Annotation: Machine learning algorithms can provide initial labels for data, which human annotators can then verify and refine.
- Crowdsourced Labeling: Using platforms that leverage multiple annotators can speed up the process while maintaining quality control.
5. Quality Assurance
Post-annotation, quality assurance processes are essential to ensure the labeled data's integrity. Implementing a review mechanism, such as a double-check system or utilizing metrics and KPIs to assess labeling quality, is critical.
6. Data Utilization
Once the data is correctly labeled and verified for accuracy, it can be fed into machine learning models. The outcome of this training will significantly depend on the quality of the annotations made earlier.
Choosing the Right Data Annotation Tool
In today’s fast-paced technological landscape, employing a high-quality data annotation tool is vital. At Keylabs.ai, we offer a sophisticated data annotation platform designed to facilitate the effective labeling training data process.
Features of Keylabs.ai
Our platform is equipped with a myriad of features:
- User-Friendly Interface: Simplified navigation ensures both novice and expert users can manage data annotation tasks efficiently.
- Customizable Annotation Projects: Tailor the platform to meet your specific dataset requirements and objectives.
- Collaboration Tools: Facilitate teamwork with built-in communication tools to discuss annotations and validations.
- Advanced Machine Learning Integration: Seamlessly connect your labeled datasets with machine learning models for immediate use.
- Review & Feedback System: Users can provide real-time feedback on annotations, promoting continuous improvement.
Best Practices for Labeling Training Data
To ensure the best results, follow these best practices:
Define Clear Objectives
Understand what you’re aiming to achieve with your data annotation project. Establish measurable goals to guide your efforts.
Invest in Quality Training for Annotators
Ensure that your annotators are well-trained to comprehend the complexities of the data they are working on. This investment pays off in the form of high-quality results.
Utilize Software Tools
Employing software tools like Keylabs.ai can significantly enhance the annotation process. Automate repetitive tasks to save time and reduce human errors.
Continuous Improvement and Iteration
Data labeling is not a one-off task. Continuously assess the quality of your labeled data and iterate on your processes based on feedback and performance metrics.
Common Challenges in Labeling Training Data
Like any process, labeling training data comes with its own set of challenges. Here are a few common ones and how to tackle them:
- Ambiguity in Data: Some data might not be straightforward. To mitigate this, ensure your annotation guidelines are detailed and revisit them frequently.
- High Volume of Data: For large datasets, consider using a mix of automated and human annotation strategies to meet deadlines without sacrificing quality.
- Maintaining Consistency: Regular training sessions and the development of clear guidelines can help maintain consistent quality across annotations.
The Future of Labeling Training Data
As AI technology continues to evolve, so too will the methods and tools for labeling training data. Here are some trends to watch out for:
1. Automation and AI Assistance
More sophisticated AI tools will emerge to assist in the annotation process, allowing for faster and more accurate labeling.
2. Active Learning
Active learning strategies will become more prevalent, where models are trained iteratively to improve their performance on datasets, thus streamlining the labeling process.
3. Enhanced Collaboration Tools
Platforms like Keylabs.ai will continue to enhance collaboration features, allowing teams across the globe to work effectively on data annotation projects.
Conclusion
Labeling training data is a crucial step in the AI and machine learning workflow. By optimizing this process and utilizing advanced tools like Keylabs.ai, businesses can significantly enhance their model performance and achieve superior outcomes. As data becomes increasingly central to decision-making, mastery in labeling training data will distinguish leading companies in the field.
Make the leap today; transform your data annotation projects with Keylabs.ai and harness the true power of well-labeled training data!